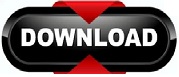
Barbedo et al., 2015 Barbedo J.G., Tibola C.S., Fernandes J.E.M., Detecting Fusarium head blight in wheat kernels using hyperspectral imaging, Biosyst.Bagheri et al., 2018 Bagheri N., Mohamadi-Monavar H., Azizi A., Ghasemi A., Detection of Fire Blight disease in pear trees by hyperspectral data, European J.Bagheri, 2020 Bagheri N., Application of aerial remote sensing technology for detection of fire blight infected pear trees, Comput.Backoulou et al., 2011 Backoulou G.F., Elliott N.C., Giles K., Phoofolo M., Catana V., Mirik M., Michels J., Spatially discriminating Russian wheat aphid induced plant stress from other wheat stressing factors, Comput.Adelabu et al., 2014 Adelabu S., Mutanga O., Adam E., Evaluating the impact of red-edge band from Rapideye image for classifying insect defoliation levels, ISPRS J.

Abdulridha et al., 2019 Abdulridha J., Ehsani R., Abd-Elrahman A., Ampatzidis Y., A remote sensing technique for detecting laurel wilt disease in avocado in presence of other biotic and abiotic stresses, Comput.The research results prove the feasibility of UAV multi-spectral remote sensing images to detect apple fire blight. For healthy samples, slightly infected samples and seriously infected samples, the omission error and commission error were 0, 7.69%, 13.34%, 7.14% and 9.09%, 0, respectively. The Kappa coefficient of the RF model is 0.904, which is the highest among all models, and the omission error and commission error are also the smallest. The results show that the overall accuracy of the RF model reaches 94.0%, which is 6.0% and 10.0% higher than the SVM model and the decision tree model, respectively. The samples with the abnormal values removed were used as input to construct apple fire blight detection models using decision trees, Random Forest (RF) and Support Vector Machine (SVM) classification algorithms, respectively. And then we use the isolation forest (iForest) algorithm to detect the abnormal values. Then, based on the Minimum Redundancy Maximum Relevance (mRMR) algorithm, we selected the Ratio Vegetation Index (RVI), Anthocyanin Reflectance Index (ARI) and Triangular Vegetation Index (TVI) from 20 candidate vegetation indices as the optimal feature combinations. In this study, we used the DJI Matrice 200 multi-rotor UAV equipped with the MicaSense RedEdge-M multispectral camera to obtain the multispectral images of apple tree canopies. It is urgent to detect the severity difference and spatial distribution of its infection in time and accurately. Apple fire blight is a common disease that seriously damages the growth of apple trees.
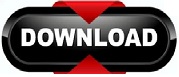